Press Downloads
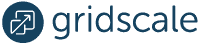
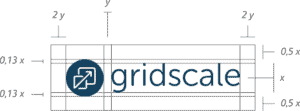
Dos & Don’ts
For us the gridscale logo is an important part of our brand. Therefore we wrote down some Dos and Don’ts how to use the Logo. Our PDF will answer all the question you may have.
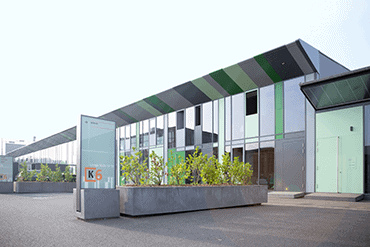
Photo Package
Here you can get a picture of us. In our package you will find photos and graphics about gridscale, the management and our products. You can use the material for editorial purposes, but you have to mention the source. Printing and publication are free of charge, but we ask for a short notification to gridscale@piabo.net. All copyrights are held by gridscale GmbH.
Press Contact
