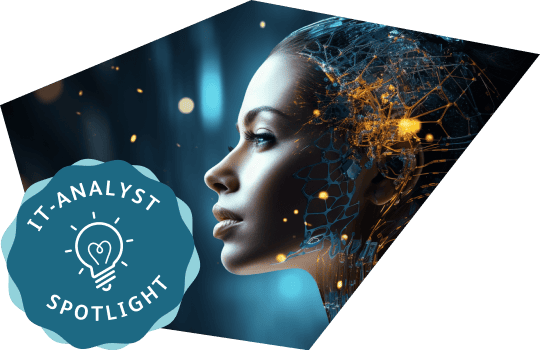
Edge AI:
Edge computing + artificial intelligence = the next level of digitalization
Companies that want to exploit the potential of artificial intelligence should also look into edge computing. The interplay between edge computing and artificial intelligence – edge AI for short – offers the opportunity to implement automation solutions across the board and thus reach the next level of digitalization. The fourth part of our analysis series on “Edge computing: between heaven and earth” discusses how this interaction works in practice and which use cases arise from it.
Beyond ChatGPT & Co: Edge AI as a growth driver
Just one year has passed since ChatGPT became publicly available and the potential of AI-based applications was brought to the attention of the general public. With the help of artificial intelligence (AI), it is ultimately possible to generate new content from available knowledge quickly, easily and in a targeted manner. From professional copywriting and software programming to customer service – the possible applications are diverse and the savings potential enormous. In fact, there is hardly a company today that is not thinking about how AI can be used effectively for its own purposes.
However, those responsible for identifying AI application scenarios should be aware that the potential of deep learning technologies extends far beyond ChatGPT & Co – especially if they are operated via an edge computing infrastructure. The interplay between edge computing and AI offers the opportunity to automate core processes across the board, i.e. outside of controlled environments, and to implement data-based business models. A huge productivity lever.
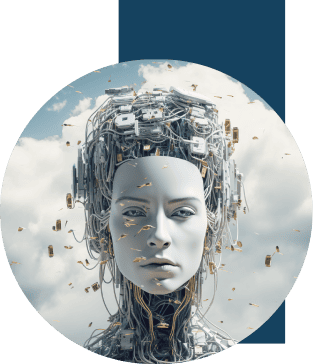
Source: market.us
This is precisely why more and more analysts and market researchers – such as Allied Market Research, Grand View Research, MordorIntelligence, MarketsandMarkets, Market.us (see illustration) and many others – are viewing the combination of edge computing and artificial intelligence, or edge AI for short, as a market segment in its own right, to which they attribute immense growth potential.
Deep learning enables automation in the field
But what makes Edge AI so attractive? Let’s start from the beginning: Conventional automation technologies have always reached their limits when the information input cannot be precisely described, specified or predicted. Deep learning programs, on the other hand, are now able to process large amounts of unstructured information and learn how to process it using pattern recognition. They can also be used flexibly, as neural AI networks are not trained to answer a specific question, but a specific type of question.
AI technologies therefore also make it possible to digitally map processes in a living environment in which a variety of factors interact (sometimes chaotically) and whose effects cannot be predicted in detail. Such living systems include road traffic as well as the human organism, the diverse interactions in a supermarket or the acreage on a farm. This opens up scope for numerous innovative application scenarios that can be realized with AI support. These range from autonomous vehicles to the dynamic monitoring and control/management of supermarkets, buildings, production facilities, energy grids, animal farms or cultivated areas through to the (early) detection of diseases in medicine.
The use of AI opens up a whole new spectrum of possibilities for automation in the field.
More and more companies already have big data
There is already plenty of data available for feeding AI systems. After all, the remeasurement of our environment has long been in full swing. Today, cameras and sensors are omnipresent, increasingly sophisticated and easily affordable. As a result, most companies today have long had a considerable (often untouched) treasure trove of data at their disposal. In fact, according to Statista, the amount of digital data generated doubled between 2020 and 2023 – and has even tripled or quadrupled since 2019 and 2018 respectively; the trend is still rising sharply.
Source: statista
Edge computing as a door opener for the realization of data-based business models
The challenge in realizing the application scenarios outlined is therefore less about generating the mass of data to feed the AI systems and more about processing it promptly and effectively. This is where the cloud model reaches its limits – and edge computing comes into play.
Why? In fact, a purely cloud-based IT infrastructure is highly unsuitable for AI-based automation on a large scale. Firstly, stable network coverage with sufficient bandwidth, which would be necessary for the prompt processing of mass data in a centralized cloud data center, is often simply not guaranteed. And even if this were the case, the transfer of mass data to the cloud would entail immense costs due to the high bandwidth required. In addition, there are even greater latencies (delays) in data transfer to and from the cloud, which would simply not be tolerable for real-time applications such as autonomous driving or the control of machines and systems.
The majority of the AI application scenarios outlined would not be technically and/or economically feasible with a pure cloud model.
The situation is different with a distributed architecture, where computing resources are provided on servers or devices at the edge of the network – i.e. at the edge. In this case, the AI applications are operated exactly where the applications are located. In this way, the stability, availability and performance of AI applications can be significantly increased and the associated connection costs and latency times minimized compared to cloud operation. In addition, edge-based application operation also facilitates the implementation of data protection requirements (privacy).
Edge computing allows AI-based automation solutions to be implemented in a technically and economically viable way across the board. Edge AI thus provides the fuel for the next stage of digitalization, which focuses on the implementation of data-based business models and the end-to-end automation of core processes.
Cloud computing is an integral part of Edge AI
Will this make cloud computing obsolete? Not at all! After all, an integral part of every AI solution is its continuous training and improvement – with the training process, i.e. deep learning, ideally taking place in a centralized data center. To train the AI model, “problem cases” from AI operations at the edge are usually uploaded to the cloud and processed there. The improvements made to the AI model in this way will flow back to the AI applications at the edge with the next update.
The edge AI model provides for constant feedback between the edge and cloud computing, as a result of which the AI model is continuously improved.
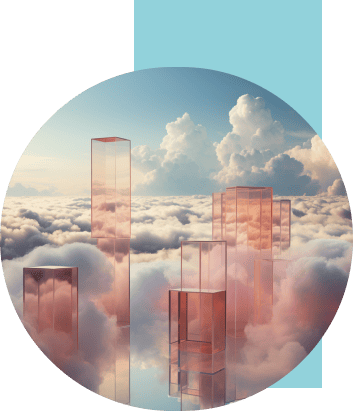
Of course, there is no such thing as THE edge AI architecture. Where the AI routines and computing resources are actually installed at the edge, whether on a device, sensor or a server built for edge operation, depends on the specific use case. It is also conceivable that individual AI applications could be operated directly in the cloud – with selected data that has been pre-sampled and pre-processed at the edge. Whether the edge computing resources work as a quasi-autonomous system or take over the pre-processing of the data in conjunction with the cloud depends on the specific use case. However, it is also clear that the applications outlined above cannot be realized without computing resources at the edge.
Conclusion: Considering (and planning) edge computing and AI together
This article shows that edge computing and artificial intelligence have immense potential together. The interplay between these two technologies opens up scope for the realization of numerous innovative application scenarios, resulting in the end-to-end automation of business processes and the implementation of data-based business models. Those responsible in companies should take this discussion as an opportunity to seriously examine the potential of the two technologies in combination.
Finally, a possible implementation of Edge AI applications should also be included in the planning of the infrastructure. The next stage of digitalization relies on the efficient interaction of cloud and edge computing resources in a hybrid infrastructure. However, this can only succeed if the hybrid cloud solution guarantees standardized management and allows flexible load distribution. In other words, the selection of a suitable hybrid cloud management platform is critical to success.
Further information:
gridscale publications on the topic
- Edge Computing: Between heaven and earth (Part 1: Introduction)
- Edge Computing: Between heaven and earth (Part 2: Architecture)
- Edge Computing: Between heaven and earth (Part 3: Practice)
- Edge Compendium: The ten most important questions
- Data Economy (Analyst Spotlight)
Third-party articles on the subject worth reading
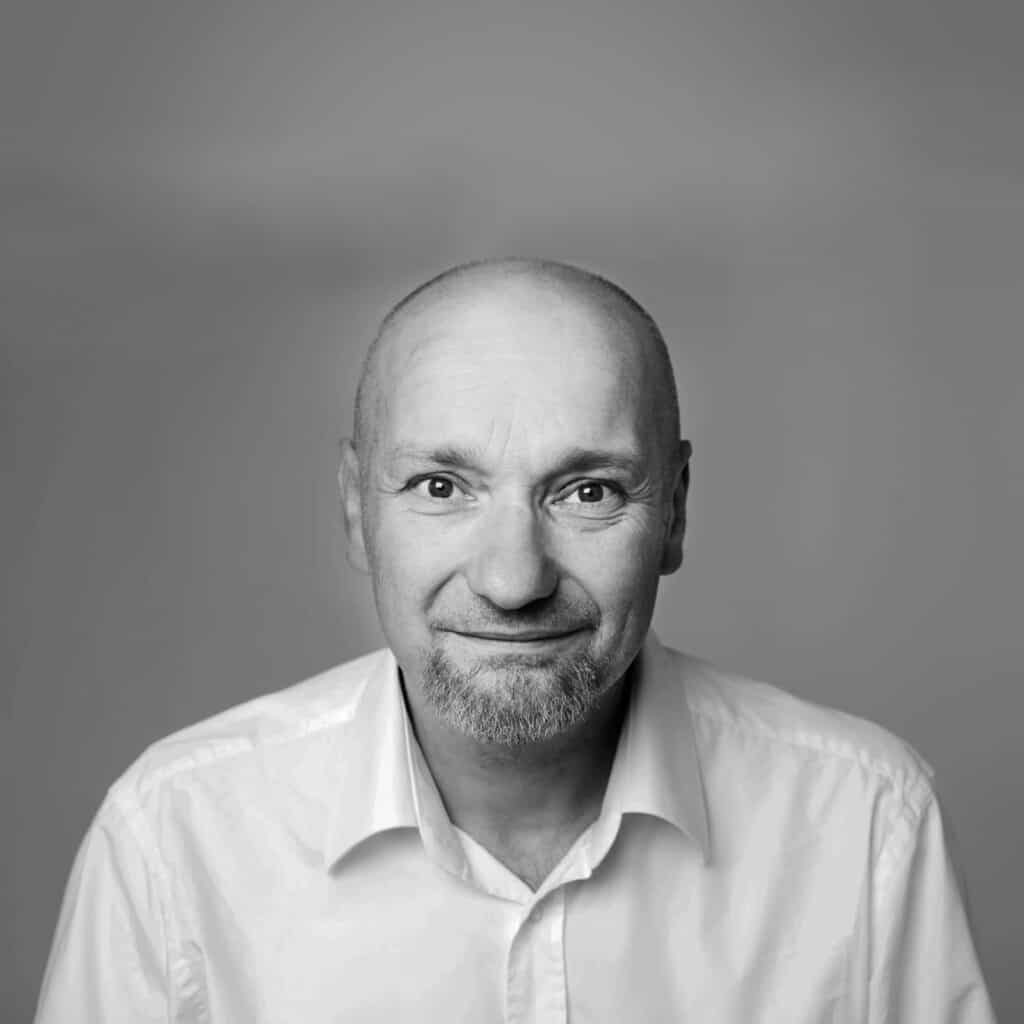
Dr. Andreas Stiehler
Dr. Andreas Stiehler accompanies as IT analyst, author and consultant
research and consulting projects for more than 20 years
digital change. His core topics here are Digital Work &
Digital Workplace, customer service in the digital transformation and the
Management of knowledge work(s). The economist with a doctorate
with a focus on behavioral economics is committed to this,
to take a holistic view of the digital transformation and
to focus more on people.
Profile on LinkedIn