How the public cloud can unleash AI innovations
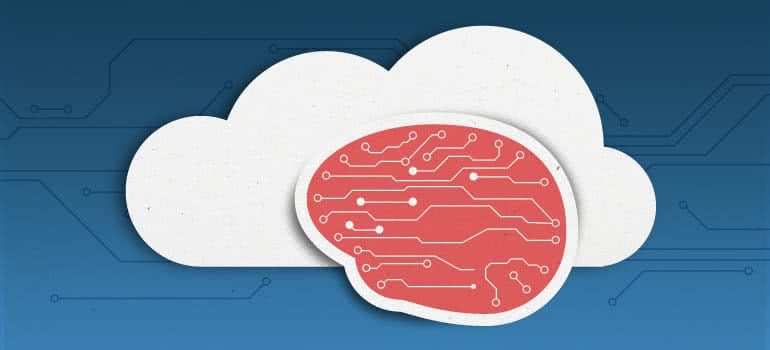
Artificial intelligence (AI) is one of the top topics in the technology sector worldwide. This is particularly evident in the market development. According to the study “AI in Europe – Ready for Take-off” by the European Information Technology Observatory (EITO), the European AI market is expected to grow to ten billion euros. In addition to hardware and software manufacturers, service providers in particular, including cloud providers, could benefit from this.
It’s like almost every hype topic. Studies are springing up like mushrooms and usually they are overflowing with figures about the best market prospects. It’s no different when it comes to the topic of artificial intelligence. According to the EY study “Artificial Intelligence in Europe – How 307 Major Companies Benefit from AI”, 72 percent of company managers are involved with AI.
In Europe, 41 percent would be in concrete AI pilot phases and 31 percent have even already integrated AI into individual or several business processes. They use a wide range of marketable AI solutions for machine learning, speech and image recognition or solutions for chat offers for customer consulting.
KI Botteneck: Ressources, IT, personnel
Despite all the euphoria, another study pours a little water into the wine. For example, a study by IDC in mid-2019 found that only about 30 percent of companies achieve a success rate of 90 percent in AI projects. Most reported error rates of 10 to 49 percent. Why do AI projects fail?
More than a quarter lack qualified personnel and unrealistic expectations of the technology. In another quarter, projects lack the necessary data or the data quality is insufficient. What’s more: AI projects pose new challenges for IT. They require a lot of computing power, which can be achieved with more CPUs, GPUs, DSPs and other accelerators. But computing power costs money. And when companies are at the beginning of their AI career, investments in hardware and software are fraught with risk for financial reasons.
“For small and medium-sized companies, building high-performance and scalable AI systems can quickly become an expensive affair.
After all, the training of algorithms and the subsequent operation of the corresponding analytics systems requires enormous amounts of computing power. In your own cellar, server room or data center, it is impossible to provide the required computing power in time and with pinpoint accuracy. Which, by the way, is usually no longer needed afterwards,” says Gartner analyst Rene Büst.
AI from the private, public or hybrid Cloud?
The alternative: AI from the cloud. But from which cloud? Private, public or hybrid. According to the IDC study “Artificial Intelligence in Germany 2019”, 61 percent of the companies surveyed use cloud-based AI services because the cloud provides IT resources in line with demand. 43 percent of the companies surveyed prefer the private cloud variant for operating the AI platform.
Among other reasons, this is for reasons of data security, which they miss in a public cloud. A study by Bitkom Research and KPMG confirms these figures. According to the “Cloud Monitor 2019” only two percent of the companies surveyed use the public cloud for AI projects. At least one quarter are planning or discussing the use of the public cloud.
Why is public cloud more suitable for AI and machine learning initiatives than private cloud? And how exactly can the company migrate operations smoothly and without too much disruption? Compared to private cloud and on-premise configurations, public cloud is much more flexible. This flexibility results in an almost unlimited degree of adaptability.
Public cloud usually offers small and medium-sized companies in particular a higher level of security and controlled access than their own data center. And with German and European public cloud providers that operate their data centers in Germany or Europe, users are also on the safe side when it comes to data protection requirements.
Custom-fit combination of CPUs and GPUs
The two critical pillars of AI and machine learning are the data as input for models and the calculation based on statistical algorithms. These data exist in different forms: relational databases, unstructured data stored as binary objects, notes in NoSQL databases or raw data from data lakes. The complex calculations require a suitable combination of CPUs and GPUs. In the current context GPUs are more expensive than CPUs. Cloud vendors offer clusters of GPU-supported VMs and containers via a pay-by-use model.
Additional computing services such as batch processing, container orchestration and serverless computing are used to parallelize and automate machine learning tasks. Public cloud providers provide APIs and services. Cognitive computing APIs such as vision, speech, translation, text analysis and search are available for developers. They can be easily integrated into applications with just one API call.
Preconfigured VM Templates
In addition to offering APIs and infrastructure, cloud vendors also offer AI tools. These tools are closely integrated with the data and computing platform. Because setting up and installing the right data science environment is complex, cloud vendors offer preconfigured VM templates.
For newcomers to data processing, there are wizards and tools that abstract the complexity of machine learning models. Because the efficiency of a machine learning model depends heavily on data quality, public cloud vendors offer data preparation tools that perform the task of extracting, transforming, and loading (ETL).
Support for live migration of virtual machines is also an important feature for companies that may already have started to implement AI. This feature ensures that internal systems run smoothly as data is transferred from an organization’s existing infrastructure to the cloud. In addition, public cloud vendors, such as gridscale, support when data needs to be moved from a private cloud or local system into the cloud.
With the exponential growth of AI, it is now time for organizations to lay the groundwork in the form of a public cloud. As the most cost-effective option for organizations seeking support for their AI technologies, the public cloud is the most efficient option. By freeing up budgets invested in physical data centers and traditional infrastructures, organizations can invest them in AI expertise.